The AI illusion: Why most companies fail and how to succeed

Artificial intelligence (AI) is heralded as a game-changer for businesses, promising revolutionary efficiency, innovation and transformation. Yet, reality paints a different picture: a vast majority of AI projects fall short of expectations. Companies frequently find their AI investments stuck at the proof of concept (POC) stage—an early trial phase designed to assess feasibility.
Rather than becoming powerful business drivers, these initiatives often drain resources without yielding meaningful outcomes. As a result, executives grow skeptical of AI’s ROI, while teams struggle to secure specialized talent and implement costly infrastructure upgrades.
Understanding the AI Illusion
The AI Illusion emerges when organizations overestimate AI’s short-term capabilities and treat it as a universal solution to complex challenges. When expectations collide with reality, blame often shifts to the technology itself rather than the flawed approach. This cycle leads to wasted resources, demoralized teams, and opportunities slipping through the cracks.
From my experience working with Silicon Valley leaders, global leading technology developers and forward-thinking enterprises from around the globe, my team and I have identified recurring pitfalls that perpetuate the AI Illusion.
First of all, most business leaders are treating AI as if it functions like traditional software. They also overestimate progress, achieving 80 percent completion rapidly but struggling to reach full deployment. And on top, they are failing to design systems that play to AI’s strengths while compensating for its weaknesses.
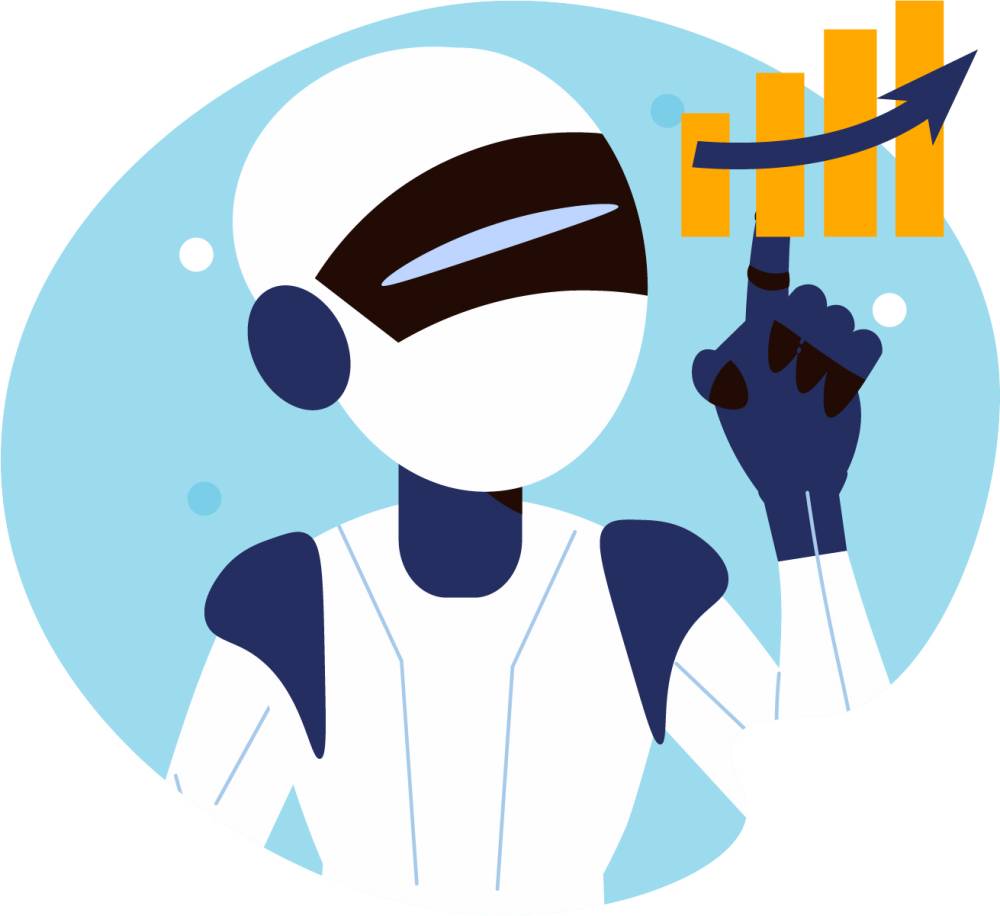
Common causes of AI pilot failures
Most POCs never reach production. To break free from this pattern, you need to confront the root causes of failure and rethink your AI strategies.
1. Undefined success metrics
One of the most prevalent and avoidable reasons AI projects fail is the lack of a clear definition of success. Many initiatives proceed without specific, measurable goals, leaving teams unable to gauge whether the technology is delivering value, such as cost reductions or operational improvements.
Solution: Define success metrics upfront, tying them directly to tangible business objectives like improved productivity or enhanced customer satisfaction.
2. Insufficient expertise
Many organizations lack the specialized knowledge required to implement and manage AI systems effectively. Hiring skilled AI professionals is both competitive and costly, and attempting to upskill existing staff often falls short of the expertise needed for successful deployment.
Solution: Forge partnerships with experienced AI consultants and prioritize training initiatives to build foundational knowledge across teams.
3. Poor data quality
AI systems thrive on high-quality data, yet many companies attempt to deploy them with fragmented or inconsistent datasets. This often results in systems that perform poorly when scaled beyond the initial trial phase.
Solution: Implement robust data governance practices to ensure clean, reliable, and accessible datasets before launching AI initiatives.
4. Prohibitive costs of LLMs
Large Language Models (LLMs): AI systems trained on extensive datasets to generate human-like responses—are frequently used in POCs. However, scaling these models can be prohibitively expensive and inefficient, especially when they lack the specialization required for specific use cases.
Solution: Prioritize finetuned models tailored to your business’s unique challenges, delivering cost-effective and targeted solutions.
5. Trust and compliance challenges
AI adoption often falters due to a lack of trust and concerns around compliance. Issues such as hallucinations or unpredictable outputs can erode stakeholder confidence and create regulatory risks.
Solution: Build transparent systems with rigorous testing and validation processes to ensure reliability and accountability.
Strategies for escaping the AI Illusion
Organizations must adopt a balanced approach that combines AI’s strengths with human oversight. Here are four strategies to help businesses succeed:
1. Tailored solutions over generic models
General-purpose AI models may seem appealing, but they’re often ill-suited for specific business applications. Tailored, fine-tuned models designed for targeted use cases deliver far better results at lower costs.
Example: In the financial sector, customized AI for fraud detection can identify nuanced transaction anomalies more effectively than a generic language model.
2. Human-AI collaboration
AI should augment human decision-making, not replace it. Integrating human oversight ensures AI systems remain aligned with business goals and builds trust across stakeholders.
Example: Customer support AI can analyze large volumes of inquiries to predict trends, while humans verify and refine responses for greater accuracy.
3. Flexible, modular infrastructure
Rigid, one-size-fits-all systems hinder AI adoption. Businesses need adaptive architectures that support multiple models and workflows tailored to specific challenges.
Example: A modular infrastructure enables seamless integration of fraud detection, predictive analytics, and customer segmentation systems, streamlining operations.
4. Continuous improvement
AI systems require ongoing refinement to stay relevant. Establishing a feedback loop allows organizations to adapt to new data, improving model accuracy and maintaining competitive advantage.
Example: An ecommerce company regularly updates its recommendation engine based on customer preferences, leading to increased sales and user satisfaction.
5 to Thrive: Steps to AI success
Take this example: A global retailer initially approached AI with unrealistic expectations, hoping for an immediate solution to inventory forecasting challenges. Early efforts faltered due to inconsistent data and a lack of human oversight. By shifting to a finetuned model and integrating expert input at key decision points, the company reduced inventory costs by 30 percent and significantly improved customer satisfaction.
You, too, can transform AI from a frustrating illusion into a powerful driver of innovation and success by following these five steps:
- Define clear success metrics: Ensure leadership aligns on measurable success criteria tied to concrete business outcomes.
- Invest in specialized talent: Engage experienced professionals and outside experts through hiring or consulting partnerships, and train your teams to support AI efforts effectively.
- Build strong data foundations: Prioritize clean, consistent and accessible datasets to power accurate AI models.
- Think beyond POCs: Design scalable initiatives that address specific challenges, focusing on cost efficiency and long-term value.
- Prioritize trust and transparency: Build systems that stakeholders can trust, ensuring compliance and reliability through robust testing and validation.
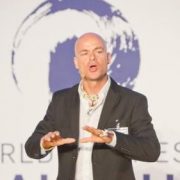
Tom Oliver, a “global management guru” (Bloomberg), is the chair of The Tom Oliver Group, the trusted advisor and counselor to many of the world’s most influential family businesses, medium-sized enterprises, market leaders and global conglomerates. For more information and inquiries: www.TomOliverGroup.com or email Tom.Oliver@inquirer.com.ph.